Unveiling Human Emotions: Exploring Image-Based Emotion Recognition through Facial Analysis
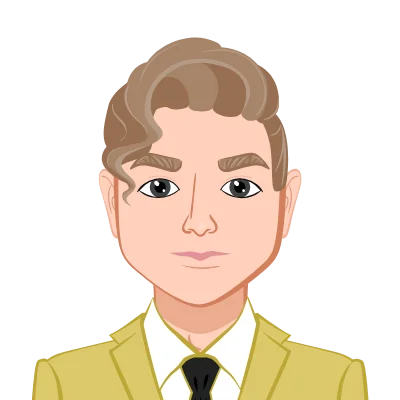
Emotion Recognition: Understanding the Basics
Emotion recognition, which is a fascinating field of study, offers interesting new perspectives on the complex world of human feelings. Examination of a person's facial expressions by researchers enables them to glean significant information about the emotional state of the subject being studied.
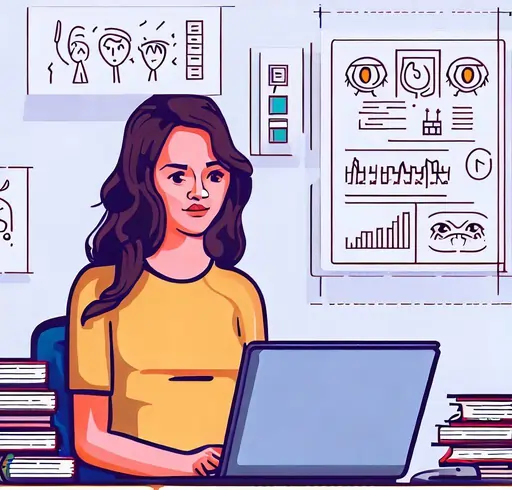
This fundamental understanding forms the basis for image-based emotion recognition that is based on facial expression analysis. Following this, we will delve into the fundamental theories and concepts that underpin the ability to recognize emotions. This will lay a solid groundwork for us to build upon as we proceed to investigate in greater depth the strategies and methods that are utilized in this fascinating field. This will allow us to move forward with confidence. Whether you are a student looking for assistance with your MATLAB assignment or an inquisitive mind exploring the wonders of computer vision, the information that has been presented here will serve as an extremely helpful resource for you.
- What is Emotion Recognition
- The Significance of Emotions in Human-Machine Interaction
- Challenges in Emotion Recognition
Facial expression analysis is used in the fascinating process of emotion recognition, which aims to recognize and comprehend the range of human feelings that can be conveyed through facial expressions. Researchers are able to decipher the complex language of emotions that are encoded in these visual cues by carefully examining facial features such as the position of the eyebrows, eye movement, and the shape of the mouth. This in-depth analysis enables the determination of the corresponding emotional state, which in turn enables computers to gain useful insights into the workings of human emotions. With this knowledge, machines are able to react appropriately in a variety of settings, whether it's modifying their behavior to provide more empathic support, enhancing human-computer interaction, or contributing to the advancement of fields such as psychology and market research. The ability of machines to comprehend and interact with human emotions can be unlocked through the use of emotion recognition, paving the way for interactions between humans and technology that are more meaningful and responsive.
Emotions wield a tremendous amount of power in the process of shaping human communication, which in turn influences our behavior, the way we make decisions, and our overall well-being. In light of the significance of feelings, scientists are working to develop computer programmed that are capable of comprehending and reacting to various expressions of emotional state. This is done with the goal of bridging the gap between human and machine interaction. It is possible that machines that are aware of their users' emotions will revolutionize user experiences by providing interactions that are personalized and empathic. In addition, these systems can make a contribution to the analysis of mental health, which helps in the early detection and intervention of problems. Emotion recognition technology paves the way for a future where machines understand and respond to our emotional states. This will foster deeper connections and enrich the ways in which we interact with technology. It does this by enabling more empathetic human-computer interfaces.
In spite of significant technological advancements, recognizing emotions based on facial expressions is still a difficult task due to the many different factors involved. One of the most significant obstacles is the inherent subjectivity and variability in human emotions, which can be seen in the fact that people's facial expressions can differ from one another and from culture to culture. In addition, aspects such as lighting conditions, occlusions, facial disguises, and partial expressions have the potential to infuse the data with noise and ambiguity. Moreover, accurately capturing subtle and complex emotional nuances, such as micro-expressions and temporal dynamics, presents additional challenges. This can be a challenge because it can be difficult to distinguish between the two. In the field of emotion recognition from facial expressions, one of the most active areas of research continues to be the development of algorithms that are both robust and reliable, able to overcome these challenges and generalize across a wide range of populations.
- Subjectivity: Since emotions can be experienced differently by different people and can also be influenced by factors such as culture and circumstance, accurately identifying them can be a challenging endeavor.
- Ambiguity: It is possible for facial expressions to be ambiguous, with certain expressions being able to overlap with one another or convey multiple emotions all at once.
- Data Variability: The performance of emotion recognition algorithms can be adversely affected by factors such as lighting conditions, head pose, occlusions, and variations in image quality.
Image-Based Emotion Recognition Techniques
Researchers have developed a wide variety of methods and algorithms in order to address the challenges that are associated with emotion recognition. In this section, we will go over some of the more common techniques for conducting facial expression analysis as a means of image-based emotion recognition. Methods such as support vector machines (SVM), convolutional neural networks (CNN), and deep learning architectures are examples of machine learning approaches that fall under this category. For the purpose of identifying distinguishing characteristics of the face, feature extraction strategies such as Local Binary Patterns (LBP), Histogram of Oriented Gradients (HOG), and facial landmark detection algorithms are utilized. In addition, ensemble methods, feature fusion techniques, and data augmentation strategies are used in order to improve the performance of emotion recognition systems and make them more robust. Ongoing research continues to investigate novel algorithms and hybrid models in an effort to improve the accuracy of emotion recognition from facial expressions and make the technique more applicable to everyday life.
- Facial Landmark Detection
- Feature Extraction
- Machine Learning Algorithms
- Deep Learning Approaches
The process of identifying particular points on a face is known as facial landmark detection. These points include the corners of the eyes, the mouth, and the nose. When these landmarks are located with precision, it is then possible to extract facial features and investigate how those features have changed over time. For the purpose of facial landmark detection, various methods such as Active Shape Models (ASM) and Constrained Local Models (CLM) have been put into widespread use. CLM estimates landmark positions by combining shape and appearance models, whereas ASM relies on statistical shape models to represent the shape variations of facial landmarks. These methods allow for accurate localization of facial landmarks, which subsequently enables analysis and interpretation of facial expressions, which in turn helps with tasks such as emotion recognition and facial animation.
Emotional state can only be determined after the extraction of relevant features has been completed. The process involves extracting relevant information from facial images so that they can differentiate between a variety of feelings. The Local Binary Patterns (LBP) algorithm, the Histogram of Oriented Gradients (HOG) algorithm, and the Scale-Invariant Feature Transform (SIFT) algorithm are all popular feature extraction methods. LBP is responsible for characterizing the texture patterns in facial regions, HOG is responsible for capturing information regarding local gradients, and SIFT finds distinctive key points. These methods effectively represent facial features in a condensed and discriminative manner, which enables emotion recognition algorithms to effectively capture essential visual cues for a variety of emotions. The development of accurate and reliable emotion recognition systems is made possible by combining the aforementioned feature extraction techniques with machine learning algorithms. These systems can then contribute to applications such as affective computing, human-computer interaction, and psychological research.
When it comes to the process of training models for emotion recognition, machine learning algorithms play a critical role. To categories facial expressions and identify emotional states, supervised learning algorithms like Support Vector Machines (SVM), k-Nearest Neighbors (k-NN), and Artificial Neural Networks (ANN) have seen widespread application. These algorithms learn from labelled training data, in which facial images are associated with corresponding emotion labels, and then generalize their knowledge to accurately predict emotions in facial images of which they have not been shown. While support vector machines (SVMs) produce decision boundaries that maximize the margin between various emotion classes, k-nearest neighbors (k-NN) take into account the proximity of labelled examples. ANNs learn complex mappings between facial features and emotions by leveraging the interconnected layers of neurons that make up their architecture. Emotion recognition systems are able to achieve a high level of accuracy and effectively capture the emotional content that is communicated through facial expressions when they make use of these machine learning algorithms.
Deep learning strategies, in particular Convolutional Neural Networks (CNNs), have been particularly influential in recent years in the advancement of image-based emotion recognition. CNNs have the ability to automatically learn discriminative features from raw pixel data, which enables them to perform extremely well when analyzing facial expressions. In order to achieve state-of-the-art results in the area of emotion recognition, pre-trained models such as VGG-Face, Reset, and Inception have been fine-tuned and used in various tasks. These models take advantage of their deep layers to extract hierarchical representations of facial features. As a result, they are able to accurately capture both low-level details and high-level semantic information. The ability to generalize well and accurately predict emotions from facial images that have not been seen enables CNNs to be trained on large-scale datasets. The accomplishment of deep learning in the field of emotion recognition demonstrates the technology's potential for advancing affective computing applications and better comprehending human behavior.
Challenges and Future Directions
In spite of the significant progress that has been made, the field of image-based emotion recognition still faces a number of challenges and has many potential future directions. The need for the system to be resilient in the face of variations in lighting conditions, poses, and facial expressions across a wide range of individuals and cultures is one of the challenges. In addition, it is still extremely important to address the limitations of the datasets that are currently available, such as the limited diversity and availability of labelled data. Exploration of multimodal approaches, which combine facial expressions with other modalities such as speech and body language, is one of the future directions that will be pursued. Both the interpretability and performance of emotion recognition models can be improved with the help of recent developments in the architectures of deep learning, such as attention mechanisms and graph neural networks. Furthermore, addressing ethical considerations and privacy concerns related to the usage of facial data is essential for the responsible deployment of emotion recognition technologies. This is because ethical considerations and privacy concerns are related to the use of facial data.
- Cross-Cultural Differences
- Real-Time Emotion Recognition
- Multi-Modal Emotion Recognition
It is possible for a person's cultural upbringing to have an effect on their emotions, which can result in different facial expressions. Researchers are looking into different approaches that can help them address cross-cultural differences and improve the robustness of emotion recognition systems across a wide range of populations. In order to accomplish this, culturally sensitive models that are able to capture the nuances of facial expressions that are specific to various cultures need to be developed. In order to improve the generalizability of the models, data augmentation techniques are currently being utilized. These techniques incorporate diverse facial images of people from a variety of cultural groups. Additionally, collaborative efforts are being undertaken by psychologists, anthropologists, and computer vision experts in order to gain a deeper understanding of the cultural influences on the expression of emotions and to design emotion recognition systems that are more inclusive and accurate. These kinds of efforts aim to develop technological solutions that are culturally sensitive and take into account the wide variety of human feelings and experiences.
The ability to recognize feelings in real time is essential for a variety of applications, including interactive systems and virtual reality. The development of effective algorithms that are capable of processing facial expressions in real time and allowing for more natural human-computer interaction is currently a focus of research and development efforts. In order to accomplish this, deep learning models need to be optimized, and hardware acceleration techniques should be utilized. Real-time performance can be improved using strategies such as lightweight network architectures, model compression, and parallel processing on graphics processing units (GPUs) or other specialized hardware. Emotion-aware virtual reality, affective computing, and emotion-driven human-computer interfaces are some of the applications that can be made possible by integrating real-time emotion recognition with interactive systems. This integration also enables immediate feedback and adaptive responses, which improves user experiences and makes it possible for new applications.
It's possible that just looking at a person's face can't tell you everything you need to know about their emotions. In an effort to improve the accuracy of emotion recognition, researchers are investigating multi-modal approaches. These approaches combine facial expressions with other modalities such as speech, body language, and physiological signals. When multiple modalities are combined in this way, it is possible to conduct an analysis of emotional states that is more comprehensive, taking into account the subtle nuances that are contributed by each modality. Researchers want to be able to capture a more complete picture of an individual's emotional state, so they are attempting to incorporate data from speech intonation, gestures, the variability of heart rate, and other physiological signals. This multi-modal approach not only increases recognition accuracy but also enables a deeper understanding of emotional states in real-world contexts. This opens the door for more sophisticated emotion-aware applications and systems to be developed in the future.
Methods for Image-Based Emotion Recognition
Utilizing a wide variety of approaches and strategies is required in order to deduce an individual's emotional state based solely on their facial expressions. In the following paragraphs, we will investigate several of the methods that are typically utilized in image-based emotion recognition. From the detection of facial landmarks to the extraction of facial features and the application of machine learning algorithms, we will explore the complexities of these methods and the role that they play in the accurate recognition and categorization of emotional states. We are going to delve into the difficulties that are encountered at each stage, such as the variations in facial expressions and the extraction of meaningful features. We are able to gain insights into the complex process of emotion recognition and the potential for developing systems that are more accurate and robust if we understand the foundations and advancements in these techniques.
Conclusion
Facial expression analysis is a fascinating area of research that has a great deal of potential application in the field of image-based emotion recognition. It is possible for machines to achieve a higher level of intelligence and responsiveness in their interactions with humans if they can comprehend and analyze human feelings. The foundation of emotion recognition systems is comprised of the methodologies that are discussed in this blog. These methodologies include facial landmark detection and extraction, feature extraction, machine learning algorithms, and deep learning approaches. The future of emotion recognition holds exciting possibilities for improving human-computer interaction, mental health analysis, personalized user experiences, affective computing applications, and a variety of other domains as researchers continue to overcome challenges and explore new avenues. The development of emotion recognition technology has the potential to positively affect many facets of our lives, paving the way for human-machine interfaces that are more empathic and intuitive.