Exploring Wavelet Analysis in University Signal Processing Courses
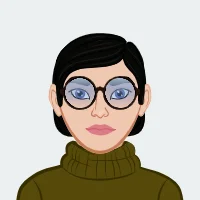
Signal processing is a captivating field that holds a pivotal role in numerous scientific and engineering applications, offering valuable assistance with Signal Processing assignment. From communication systems to medical imaging, signal processing techniques are crucial for extracting meaningful information from signals. Among the powerful tools in the signal processing toolbox, wavelet analysis stands out. In this blog post, we will immerse ourselves in the realm of wavelet analysis and unravel its significance in university signal processing courses. Delving into the intricacies of wavelet analysis not only enriches students' theoretical understanding but also equips them with practical skills, proving invaluable in assignments.
Wavelet analysis presents a unique approach by allowing the decomposition of a signal into different frequency components. This is particularly advantageous when dealing with signals exhibiting non-stationary behavior, a common scenario in real-world applications. The wavelet transform, a fundamental concept in wavelet analysis, involves convolving a signal with a scaled and translated version of a basic waveform called a wavelet. This process provides insights into the signal's frequency content at various scales, enabling a more adaptive and localized analysis.
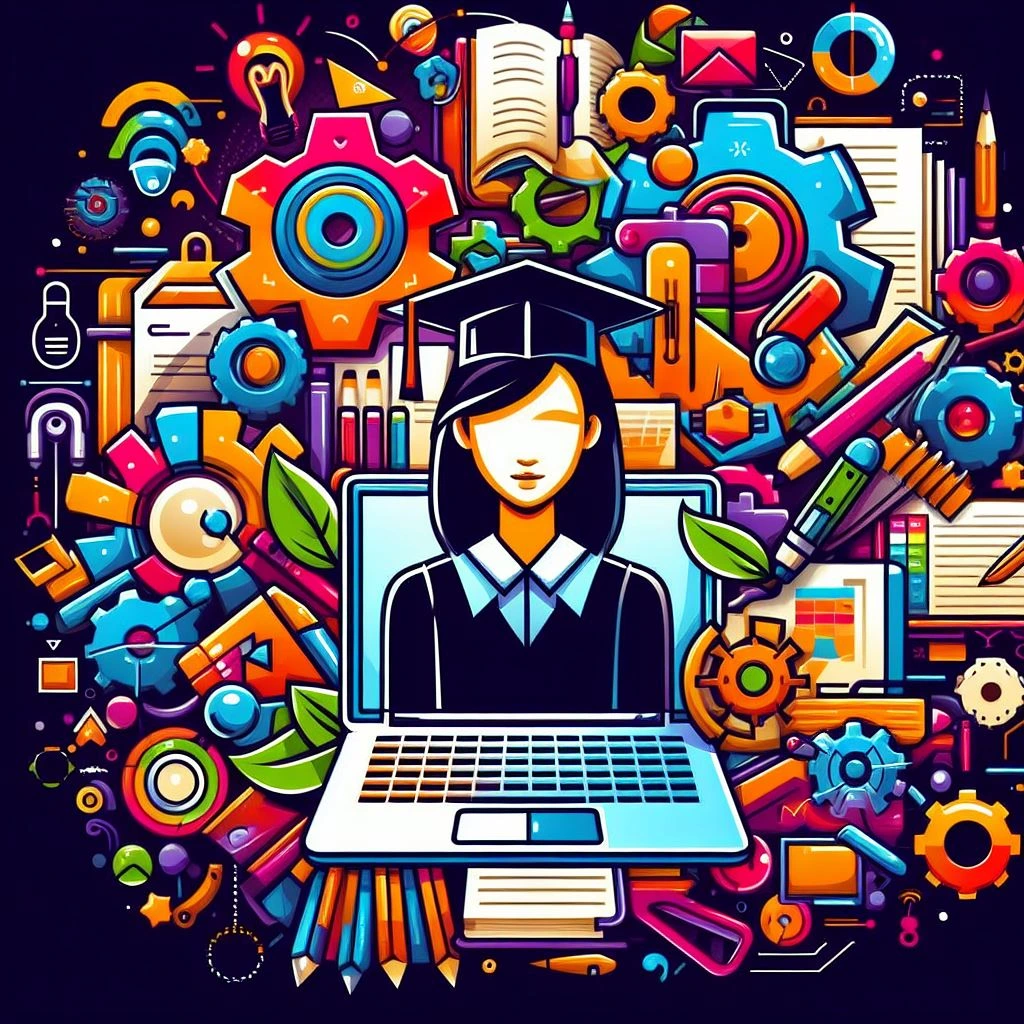
For university students tackling signal processing assignments, a solid grasp of wavelet basics is indispensable. Exploring different types of wavelet transforms, understanding how to choose the right wavelet function and scale for a given signal, and appreciating properties like orthogonality contribute to a robust foundation. This knowledge not only enhances their ability to comprehend theoretical concepts but also empowers them to apply wavelet analysis effectively when faced with practical assignments.
Unraveling the Basics of Wavelet Analysis
Wavelet analysis is a mathematical technique that allows the decomposition of a signal into different frequency components. Unlike traditional Fourier analysis, which represents a signal as a sum of sinusoidal functions, wavelet analysis captures both frequency and temporal information simultaneously. This dual representation makes wavelet analysis particularly useful in scenarios where signals exhibit non-stationary behavior.
In university signal processing courses, students often encounter complex signals with varying frequencies. Traditional methods may struggle to accurately represent such signals, leading to information loss. This is where wavelet analysis shines. It enables a more adaptive and localized analysis, breaking down a signal into its constituent parts at different scales.
One fundamental concept in wavelet analysis is the wavelet transform. This transform involves convolving a signal with a scaled and translated version of a basic waveform called a wavelet. The result of this convolution provides information about the signal's frequency content at different scales. This multi-resolution analysis is a key advantage of wavelet techniques.
Students can gain a solid understanding of wavelet basics by exploring the different types of wavelet transforms, such as the continuous wavelet transform (CWT) and the discrete wavelet transform (DWT). Learning how to choose an appropriate wavelet function and scale for a given signal is a valuable skill that empowers students to effectively apply wavelet analysis to real-world problems.
Moreover, studying wavelet properties like orthogonality and compact support enhances students' mathematical intuition. The ability to interpret results in both the time and frequency domains equips them with a versatile toolset for signal analysis.
Practical Applications of Wavelet Analysis in Signal Processing
Wavelet analysis finds applications in a myriad of signal processing tasks, making it a versatile tool for students to master. One notable application is signal denoising. In real-world scenarios, signals are often corrupted by noise, affecting the accuracy of subsequent analyses. Wavelet denoising techniques exploit the sparsity of noise in the wavelet domain, enabling effective noise reduction while preserving signal features.
Another important application is compression. In the era of big data, efficient signal compression is crucial for storage and transmission. Wavelet-based compression algorithms, such as JPEG2000 for image compression, capitalize on the energy concentration of signal information in a few dominant wavelet coefficients. This allows for high compression ratios with minimal loss of important signal details.
Wavelet analysis is also widely used in biomedical signal processing. In electroencephalogram (EEG) analysis, for instance, wavelet techniques aid in the identification of specific frequency components associated with brain activities. This is invaluable in diagnosing neurological disorders.
By exploring these practical applications, students can bridge the gap between theoretical concepts and real-world problem-solving. Assignments that involve signal denoising, compression, or biomedical signal analysis provide hands-on experience and reinforce the applicability of wavelet analysis in diverse domains.
Overcoming Challenges and Future Directions in Wavelet Analysis
While wavelet analysis offers powerful tools for signal processing, it is not without challenges. One notable challenge is the selection of an appropriate wavelet basis. Different wavelets are suited for different types of signals, and choosing the wrong one can lead to misinterpretation of results. Students should be aware of this challenge and learn to select wavelets based on the specific characteristics of the signals they are analyzing.
Another consideration is the trade-off between time and frequency resolution. The uncertainty principle in wavelet analysis states that it is impossible to simultaneously achieve perfect time and frequency localization. Students need to understand this trade-off and make informed decisions based on the requirements of the problem at hand.
Looking forward, the field of wavelet analysis continues to evolve. Advanced techniques such as wavelet packet decomposition and complex wavelet transforms offer even more sophisticated tools for signal processing. Students who grasp the fundamentals of wavelet analysis in their university courses are better prepared to explore these advanced techniques and contribute to the ongoing developments in the field.
Wavelet Analysis in Image Processing
One compelling area where wavelet analysis demonstrates its prowess is in image processing. Images are essentially two-dimensional signals, and analyzing them requires techniques that can capture both spatial and frequency information. Wavelet analysis, with its ability to provide multi-resolution representations, is exceptionally well-suited for image processing tasks.
In image compression, wavelet transforms offer a distinct advantage over traditional methods. The hierarchical structure of wavelet coefficients allows for selective compression of different frequency components, facilitating more efficient storage and transmission of images. Students exploring this aspect of wavelet analysis gain insights into widely-used image compression standards like JPEG2000, where wavelet techniques play a pivotal role.
Furthermore, wavelet-based image denoising is a critical application. Images captured in real-world scenarios often suffer from noise introduced during acquisition or transmission. Wavelet thresholding techniques can selectively remove noise while preserving the essential details in the image. Assignments in image denoising empower students to apply wavelet analysis to enhance the visual quality of images, a skill valuable in fields such as photography, medical imaging, and satellite image processing.
The exploration of wavelet-based image processing extends to applications like image enhancement and feature extraction. By understanding how wavelet coefficients capture variations in both space and frequency, students can manipulate these coefficients to accentuate specific image features or enhance overall image quality. This practical knowledge is invaluable in fields ranging from computer vision to remote sensing.
Wavelet Analysis in Audio Signal Processing
The influence of wavelet analysis extends beyond the visual realm into the auditory domain, making it a crucial tool in audio signal processing. In university courses, students encounter assignments involving the analysis and manipulation of audio signals, where wavelet techniques provide a nuanced approach.
One compelling application is audio compression. While traditional methods may result in perceptual distortions, wavelet-based compression algorithms, such as those employed in the MPEG-4 audio standard, offer efficient compression with minimal loss of audio quality. Assignments in this area can challenge students to implement and evaluate wavelet-based audio compression techniques, fostering a deep understanding of the intricate relationship between time and frequency characteristics in audio signals.
Wavelet analysis also finds application in audio denoising, where the challenge is to preserve the integrity of the signal while removing unwanted noise. Assignments in this domain can task students with implementing wavelet thresholding methods for audio denoising and exploring their effectiveness under different noise conditions.
Moreover, studying wavelet modulation techniques enhances students' ability to analyze and manipulate audio signals with varying spectral characteristics. Tasks related to identifying and isolating specific frequency components in audio signals provide a practical context for mastering wavelet analysis in the realm of audio processing.
Incorporating these applications of wavelet analysis in audio signal processing into assignments not only deepens students' understanding of the subject but also equips them with skills applicable in fields such as audio engineering, telecommunications, and speech processing.
Conclusion
In summary, the integration of wavelet analysis into university signal processing courses not only deepens students' comprehension of signal representation and processing but also empowers them with practical skills essential for tackling assignments and overcoming real-world signal processing challenges. From mastering its fundamental principles to applying them in diverse scenarios, wavelet analysis provides students with a versatile toolkit.
As technology continues to advance, the significance of wavelet analysis is poised to expand further. Its adeptness at extracting crucial information from intricate signals positions it as a pivotal topic for students aspiring to excel in the ever-evolving field of signal processing. The adaptability of wavelet analysis, evident in its applications such as signal denoising, compression, and biomedical signal analysis, underscores its relevance in addressing the complexities of modern data. Thus, students equipped with a solid foundation in wavelet analysis are not only well-prepared for current challenges but also primed to contribute to future innovations in signal processing. In essence, the inclusion of wavelet analysis in the academic curriculum serves as a strategic investment in shaping a cadre of signal processing professionals capable of navigating the dynamic landscape of technological advancements and complex signal data.